Novel approach provides faster, more accurate diagnosis for mechanical failure
By David Mitchell
It was late afternoon on Jan. 15, 2009, when every air traveler’s worst fear came to pass. Moments after takeoff, a U.S. Airways flight struck a flock of geese not far from LaGuardia Airport. Pilots Chesley “Sully” Sullenberger and Jeffrey Skiles were catapulted into a scenario you train for, but are never fully prepared.
Calling upon skills very few pilots possess, Sully, whose story has since been shared on the big screen in a movie of the same name, was able to quickly assess the failure, run through mental models of his available options, and determine that crash landing his own aircraft in the Hudson River was the only available course of action. Because of his decision making, all 155 aboard the plane were saved.
It’s a famous example of a common problem in flight scenarios: What happens when there is mechanical failure with the aircraft? In this example, passengers were lucky to have a flight crew of uncommon abilities. Many aren’t that lucky.
New research from Georgia Tech, however, could provide pilots with faster, more reliable autonomous assistance, better diagnosing problems and impacts of various decisions, and ultimately saving lives.
“Typically, when bad stuff happens, the autopilot kicks off and says, ‘have fun,’” said Matthew Gombolay, assistant professor in the School of Interactive Computing and the faculty lead on the project. “That’s the opposite of what we want. When damage occurs, the pilot needs time to re-engage, experiment with the flight controls to figure out how the damaged plane is responding, and use that information to land safely.”
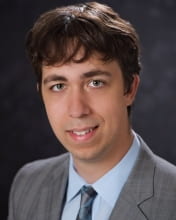
The idea of the research is that perhaps there is a better way to replicate the mental gymnastics Sully had to perform using machine learning and optimization. The system weighs the value of taking some crazy action to learn how the destabilized aircraft works after failure versus the probability that the aircraft will be able to maintain its trajectory and achieve its mission, i.e. safely landing the plane.
The system will forecast future decisions and their impact on that trajectory, allowing it to take a potentially unsafe maneuver to gain additional information about how the plane works as long as there is still high confidence it can return to a safe trajectory. This trial-and-error is essentially the type of decision-making pilots like Sully must perform in their head during emergency scenarios, offering a safer and less-permanent result.
“We want the aircraft to perform maneuvers that are both safe and will give us additional information about the failure. That is the goal of our novel algorithm ,” said Mariah Schrum, a Ph.D. student and co-author of the paper. “Our approach is faster than our baselines, but also beat our baselines in terms of how much information it gains in a time step, as well.”
“Even if the pilot takes thirty seconds to adapt to the situation – the same 30 seconds Sully had — that’s a lot of potential energy lost in an uncontrolled descent,” Gombolay added. “Those seconds could mean life or death depending on the situation.”
The hope is that, one day, autopilots onboard every commercial flight will simply flip from “nominal” operation to an “adaptation” setting similar to the one being developed by the Georgia Tech team that will safely and automatically land the plane just as well as expert pilots like Sully.
This particular study was demonstrated in simulation on a Boeing 747. The researchers are now moving forward with testing on remote control aircraft. The research could also have interesting implications in the health care space. Gombolay and Schrum are looking at a similar approach to address trial-and-error medical responses to seizures in epilepsy patients.
This research was accepted and presented at the 2020 International Conference on Robotics and Automation. This research is supported in part by the National Science Foundation(NSF) under Grant No. 1545287 This research was supported in part by the National Science Foundation(NSF) under Grant No. 1545287